THE INTRICACIES AND APPLICATIONS OF DATA SCIENCE
- liza201269
- Jan 3, 2022
- 12 min read
Updated: Jan 9, 2022
IN CONVERSATION WITH MR. SURAAJ HASIJA, DATA SCIENTIST.
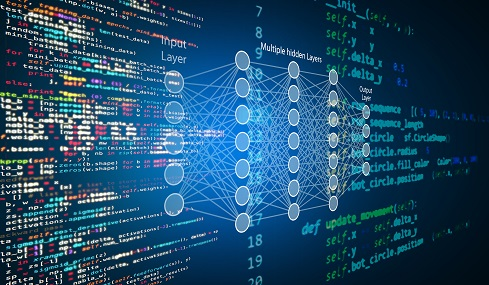
Starting his career as a co-founder of 'Googly Network', an online magazine for trending issues, Mr. Suraaj Hasija has gained vast experience in the field of Data Science. Currently working as a senior Data Scientist at Mastercard, his forte includes Data science, Statistics, Python, Finance, Advanced SQL and Machine Learning. Throughout his long and distinguished career in data science, Mr. Suraaj has worked as a data analyst at GroundTruth along with a business technology analyst at ZS.
He can be reached and contacted through LinkedIn.
Q1) Can you take us through a day in the life of a Data Scientist? What are the tasks you’re required to perform exactly on a day-to-day basis?
“One needs to ensure that the problem is clearly specified in the problem statement. This problem is then divided into various phases of a project.”
The work that data scientists do is not done on a day-to-day basis. The process starts with the stakeholder approaching the data scientist with a problem. One needs to ensure that the problem is clearly specified in the problem statement. This problem is then divided into various phases of a project. The first phase involves designing the solution by making an outline of the project. This can take a few days to few weeks after which they start getting the necessary resources for the project. Even if the project is to be conducted by a single person, they first prepare the pipeline for the data which comes from the data warehouse. This involves sanitization and manipulation of data to ensure that it is clear. After this, the data will be visualized by using Tableau, which is a very helpful tool to present the data.
Q2) What according to you is the future of Artificial Intelligence? Does your viewpoint resonate more with Mark Zuckerberg; who sees AI as a technology that can save human lives and enhance the safety of self-driving cars along with diagnosing medical conditions or with Elon Musk who sees AI as a threat to the world by eliminating jobs in the future?
“A good balance is needed between machine and human work to have an edge over others.”
I do not resonate with any of them as they are very knowledgeable people who might have their reasons to believe in what they have said. I believe AI can complement human intelligence. My favorite superhero is Ironman because of the conjunction of machine and human energy. Most Indians will resonate with Ironman because this combination brings out phenomenal results. Machines are a useful tool that makes our work easy and helps us solve our problems, but the overuse of them to do harm is dangerous. A good balance is needed between machine and human work to have an edge over others.
Q3) What is the work culture like for a data scientist? How is the work-life balance when it comes to jobs in the field of Data Science?
“You will get a better work-life balance at an established company, but the hard work in startups will be fruitful for you.”
It depends on the company that you are working with. For example, if you are working with a start-up, which is recommended in the beginning stage of your career, you will be able to explore the work as both you and the company are learning and growing together, but at the same time, it will take a lot of time like 12 to 14 hours a day. But this is fruitful at the end of that phase because you get to learn and grow a lot. When you work with an established company, they ensure that their employees have a good work-life balance. They expect you to make the timelines for the project according to your fixed working hours.
Q4) Do you believe that in the current market, be it a consulting firm or a manufacturing company, the biggest asset a company can have is data? Do you think that a strong analysis of that data could give that company leverage over its competitors? What is your take on it?
“Data is the biggest asset only when it is successful in converting your hard work into smart work. It complements the work of the people who are using it to perform their job.”
Data might not be the biggest asset for a company, but the people who are making use of that data are the biggest asset. For a manufacturing company, the raw material and the skilled labor is more valuable than data, whereas, for a management consultancy company, healthcare analytics or robotics data can be their biggest asset. Data helps a lot in converting your hard work into smart work as it is used to analyze the situation and helps in achieving efficient results. Data is the biggest asset only when it is successful in this conversion. It complements the work of the people who are using it to perform their job.
Q5) Are there enough people doing data analysis related projects for NGO’s? Do you think the power of data science can benefit non profit organizations?
“Big companies collaborate with NGO's helping them in finding the right type of customers which will help in funding the NGO's.”
Yes, there are many organizations helping Ngo’s. So there are big companies and well established Ngo’s that are working together. What they do is they help them in forecasting, help them in finding good leads where they can get funding and also helping them in making presentations which attract investors. In between a 17 year old guy and a 40 year old guy, it is obvious that a 40 year old guy would help an Ngo, because he/she has enough for his future so he/she would essentially start giving back to the community. So companies help Ngo’s in gaining this type of information and a database of these type of people which would help them in growth.
Q6) In your opinion, how can data be used to improve the quality of life? Do you think it is a powerful tool to fight the problems like poverty and climate change or is it just a field restricted to corporates?
“To solve field and area wise problems, institutions need something which will give them direction to act on, that's where data comes into picture.”
Government has data of all the citizens residing in the country. So for doing good for the poor or any community, the government should know first how much is required. Let's say Government comes to a company like Infosys to tell them about the health care centres in Delhi which are in need of development, if government had developed estimates randomly, many health care centres would have been ignored. So now when it gets the information about say the number of beds required, now the focus gets shifted to the right place with right amount.
Q7) .Since data is the future, do you believe that the field of data analysis will prove other fields of jobs redundant? Do you believe that the softwares used in data analysis can act as a substitute for Human Resources? What is your opinion on the same?
“Robots solely act on data provided whereas humans act on data as well as the intuition of the person.”
No, I don't think software can remove the involvement of human resources. machine cannot know the intent of a person, it would take many data points, but it would never be able to capture the intent of a person. A human captures the emotions as well and can grab the intent of the person from his/her gestures. We can take the example of banking industry, let's say a fraud happened and an amount of say 10k gets deducted from your account, would you be comfortable to express your problem to a robot or a person. I think we all would be wanting to express such situations to a person rather than a robot.
Q8) Can you take us through your journey from working as a Data Analyst at GroundTruth to working as a Data scientist at MasterCard?
“It doesn't matter whether you are a data analyst or a data scientist, you get similar projects with different roles.”
I still make models, it is just the change of name in designation. In Ground Truth, there was a project in which we had to focus on vendors who were giving us information about apps like Uber and Zomato. But the problem which arised was that we were paying double the amount for the same cause as there were two vendors who were giving the same type of information. When I got to Mastercard, the domain changed but the activity is still same, so at Mastercard I was on a project which was focused on the type of queries a bank asks to payment caterers, so in that case, the billing inquiries were recorded. Hence, the overall work is same even the industry changes.
Q9) How did your experience in your first data science project help you in developing your expertise in the field and what were the challenges you faced?
“People make simple things complex, there is no need of a sword when a cake can be cut with a knife.”
It is rightly said that if a cake can be cut through a knife then sword is of no need, so the data was very small ,I googled about what can we do about this, I got to know that I can do linear regression and a line of forecast can be drawn. I did this in excel on a very small scale. After that I went to learn python and I scaled it. I did not start with a titanic kind of problem, I started with a very small problem and then did many projects on the same.
Q10) What is the organisational structure for projects? Whom are you teamed up with? Is there any assessment which is carried out prior to the formation of teams for specific projects?
“It depends on the type of ask that comes from the stakeholder. If it's a one week project, we do not generally divide it into subtasks and do it. We actually jump into the analysis and try to find easier ways of doing it.”
What generally happens is that a stakeholder comes to us with a type of a problem, and this problem could be an open ended one. For example, someone comes to us and says, “I have to increase the sale of this product”. Now to increase the sale of this product, there are multiple factors. Let's say, what is the load time of your website or how the UX looks like? What is the functionality? So these are a few factors in determining the sale of the product. Therefore, to interpret the problem statement, you need to ask relevant questions to the stakeholders regarding that project. Once the relevant questions are asked, you divided into subgroups. When it is divided into subgroups, Again that process starts: designing of the solution to architecting the solution. What all tools would be required, which all people do you think have expertise in that area for. So probably if it's a very large, good project and it has very high impact, you'll call an expert who could understand that data for you. Then that person comes and interprets the data to you so you get an understanding of it, which is followed by the data exploration part. After that comes the modeling part where you analyze different models, different algorithms that could help you. Just after that, you evaluate that model, which model is working around it as well. Like why this model is working best around your data. So this is how a trivialized cycle of process looks like or a project looks like, which we actually follow, which is followed industry wide. But it depends on the type of ask that comes from the stakeholder. If it's a one week project, we do not generally divide it into subtasks and do it. We actually jump into the analysis and try to find easier ways of doing it.
Q11) In your opinion, how can practices related to data science be instilled in day to day activities to improve efficiency at ground level?
“If you take a diet plan, the dietitians ask you to buy a weighing machine because they want you to monitor your progress at a weekly level. That is why the data is important..”
Generally when a diet plan is taken, the dietitian immediately asks you to buy a weighing machine because they want you to monitor your progress at a weekly level. If you do not monitor it, you won't know about any changes in your body. But if you're constantly monitoring your weight, if you even see one kilo or two kilo movement, you'll suddenly take action. So that is what data tells. You now know how to use data science in reality. There should be a timeline. Let's say three months. There's the target that is ten kg. Now I have to again divide it into subtasks that in each month I'll lose around three kg. So how would I be able to monitor it? Like my daily calorie consumption? It could be one thousand calories, but I should be able to reduce that much calories. I'm wearing this Apple watch. It captures all the details of my activity. If my target is to reach 500 calories daily, so with that data, I'll be able to see how much I am reducing on a daily basis. So if you are able to implement this on daily level, you'll be seeing phenomenal results within yourself. That is why the data is important.
Q12) Is it a good choice for a data scientist to work at a startup at the beginning of his/her career? What would you suggest a person, just beginning in this field to start with; A Big company or a Startup?
“Personally, I would ask you to join a startup because there are immense learning opportunities. And once you see yourself growing and you see that you have gained enough and it comes a point where the saturation happens, feel free to switch.”
Starting with a startup could help you a lot, because at that time you get to learn a lot and you do not worry about the timeline since you're learning about it. So personally, I would ask you to join a startup and once you see yourself growing and you see that you have gained enough and then come a point where the saturation happens, feel free to switch. So think about what you actually want to do and what you’re like in startup. They'll give you enough opportunities to explore yourself because in startups, they do not have concrete boundaries. And also they are very open to relocation. But if you start with a big company, the growth opportunities would be limited.
Q13) With so many branches expanding rapidly and with the demand always raising the bar, Do you think it is becoming harder and harder to be just a ‘Generic Data Scientist’? Is this the reason why people are compelled to choose their niche?
“A generic data scientist is what industry needs. Industry needs a simple solution, not complex solutions to simple questions.”
I have seen many people who have defined a niche for themselves. I think there's a lot of scope currently in data science but because of the commercialization of this field, there are enough people with this skill. However, the jobs aren't enough. So to have an edge over those data scientists, people start to look for a niche just because of the industry scenario that everyone knows about linear regression. Everyone knows about logistic regression, but what to do with it, how to implement it effectively. I do not have a niche. I was not involved in modeling which is just 20% of the actual work. But the 80% of the work is preparation of that data. So if you know the industrial usage of your skill, then it helps. If you just know the course, where only the modeling is stressed, you won't be able to find the right opportunity for you. If you know what actually happens in the industry, if you know what could actually help you in landing a good job, it will help. Then there is no need to create a niche. A generic data scientist is what industry needs. Industry needs a simple solution, not complex solutions to simple questions. So just think of how you can solve a problem, not the different ways to solve one problem. So as a data scientist, 50% is interacting with the client, getting to know the problem statement better, asking the right questions and designing that solution, then the secondary part is deciding the tool or technology that you're going to use.
Q14) Can a person build a career in the field of Data Science or AI without a traditional B.Tech. degree, say with a degree in economics or mathematics? If yes, then how can the person go about self-learning the basic concepts, can you suggest some sources for the same?
“Good domain knowledge helps in analysing the data of that particular field. People who have such knowledge actually have an edge over engineers because they are able to act as subject matter experts in that field.”
Yes, one can build their career in the field of Data Science without a B.Tech. degree. People who have not done engineering have the advantage of having the domain knowledge of their particular course. For example, an economics student will know about the concepts of economics and a statistics student will have the knowledge of statistics which helps a lot in data analysis. Good domain knowledge helps in analysing the data of that particular field. You can do some courses in programming languages, excel and tableau from online sources, and then apply it to the data sets of your domain. Using this domain knowledge and data analysis, build a portfolio around it by analysing various data. People who have such knowledge actually have an edge over engineers because they are able to act as subject matter experts in that field.
The Panel Discussion, therefore, revolved around the various aspects of Data Science and included discussions on :
1)Understanding the daily life of a data scientist and the tasks they’re required to perform on a regular basis.
2) Understanding why data is one of the biggest assets, and how it facilitates hard work into smart work.
3) Explanation of decision tree and linear regression.
4) Addressing the need for utilizing the power of data science for enhancement of social startups and non-profit organizations.
5) Understanding the difference between choosing a niche and being a general data science manager.
Mr. Hasija also engaged with the audience and satisfied a number of queries with immense detail, always providing valuable insights based on his own experiences. We extend our heartfelt gratitude for such an extremely informative discussion that helped us achieve more clarity about the work of a Data Scientist. We look forward to conducting more such valuable sessions with him in the future!
Through this blog, we would also like to extend an invitation to professionals from various fields, for a conversation with us on multidisciplinary issues and themes. For further details, please feel free to contact us through our website.
Comments